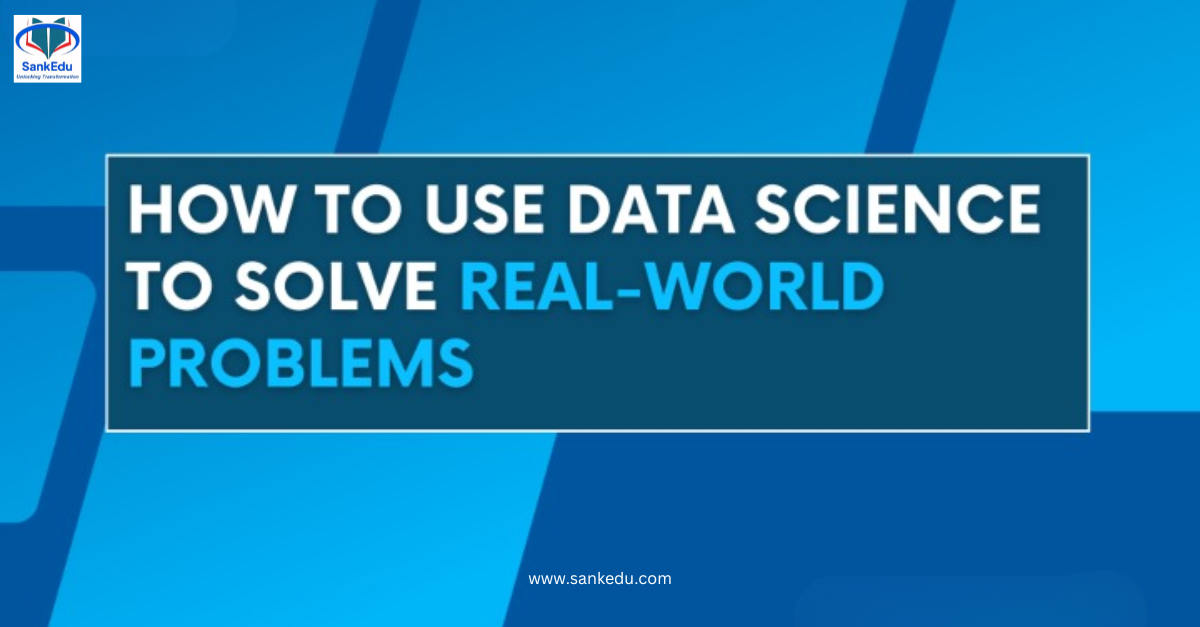
Introduction:
Data science is a powerful discipline that enables us to extract valuable insights from data,
empowering us to solve real-world problems effectively. In this blog, we’ll explore how data science
can be utilized to address various challenges across diverse domains, leading to informed decision-
making and innovative solutions.
1. Problem Definition:
The first step in any data science project is to clearly define the problem you want to solve. This involves understanding the context, setting objectives, and outlining the scope of the project.
Whether it’s optimizing business processes, predicting customer behavior, or tackling environmental issues, a well-defined problem provides the foundation for the entire data science process.
2. Data Collection:
Accurate and relevant data is the lifeblood of any data science initiative. Depending on the problem
at hand, data can be sourced from various channels, such as databases, APIs, web scraping, or even IoT devices. It’s essential to ensure data quality, address missing values, and manage data security and privacy concerns during this phase.
3. Data Exploration and Preprocessing:
Before diving into complex algorithms, data exploration and preprocessing are essential to gain a deeper understanding of the data. Visualization techniques and statistical analyses can help identify
patterns, correlations, and outliers. Data cleaning, normalization, and feature engineering are crucial
to prepare the data for modeling.
4. Model Selection:
Choosing the appropriate model is critical to the success of a data science project. Depending on the
problem type (classification, regression, clustering, etc.), various algorithms like decision trees,
random forests, neural networks, or support vector machines can be considered. Evaluating different models and selecting the one with the best performance is essential.
5. Model Training and Validation:
Cross-validation techniques can be employed to ensure robustness and avoid overfitting.
6. Predictions and Insights:
These predictions can provide valuable insights, helping stakeholders make informed decisions. For
example, in business settings, data science can aid in demand forecasting, resource optimization and customer segmentation.
7. Iteration and Improvement:
Data science is an iterative process. The first iteration might not yield perfect results, but the insights gained from it can lead to further improvements. Continuously refining models, incorporating new data and adapting to changing circumstances can enhance the effectiveness of the solution over time.
8. Communication and Visualization:
Presenting the results in a clear and understandable manner is crucial for successful implementation. Visualizations, dashboards, and interactive reports help convey complex findings to stakeholders and facilitate data-driven decision-making.
9. Ethical Considerations:
While data science offers immense potential for positive impact, it also comes with ethical responsibilities. Ensuring fairness, transparency, and privacy in data handling is paramount. Bias detection and mitigation should be practiced to prevent unintentional discrimination.
Conclusion:
Data science is a formidable tool that empowers problem-solving across various domains. By
following a structured approach, defining clear objectives, and employing ethical practices, data
scientists can effectively address real-world challenges and contribute to a more informed and data-
driven society. Remember that collaboration between domain experts and data scientists is key to
developing impactful solutions that create a positive difference in the world.
Recent Posts
Recent Comments
- Daniel Ndiema on Data-mining with Python
- Daniel Ndiema on Project 3 Basic Plotting Operations
- Daniel Ndiema on Project 1 Fundamentals of Python
- Daniel Ndiema on Project 2 Data Visualization
- Daniel Ndiema on Introduction to Python Exercises
0 responses on "How to Use Data Science to Solve Real-World Problems"