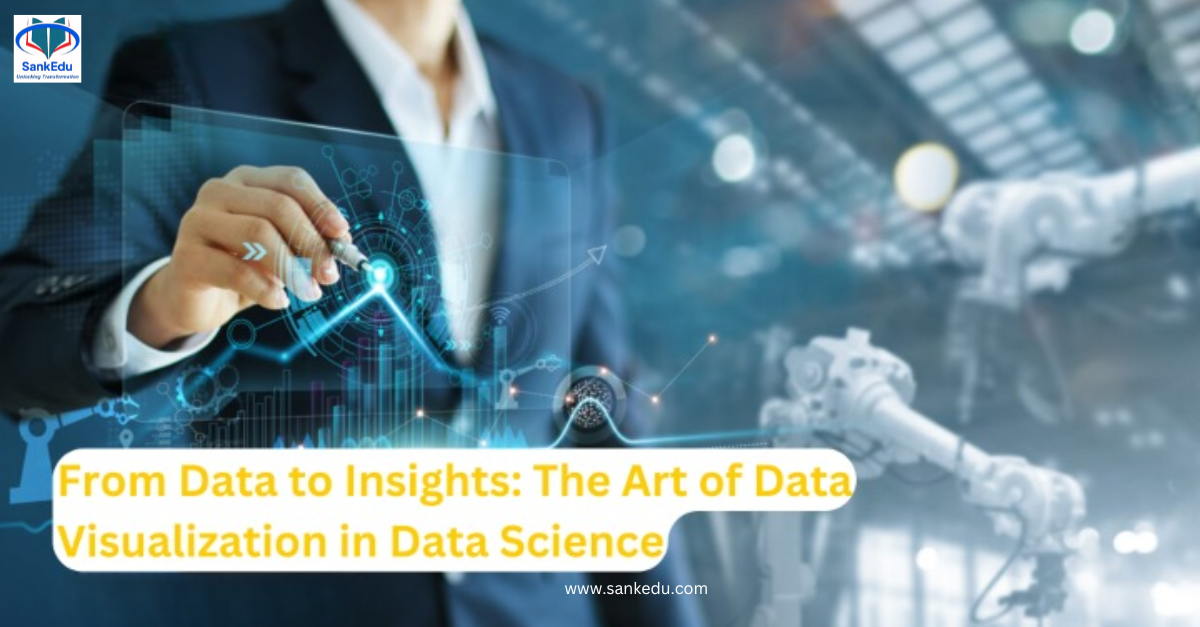
Introduction
Data science is a dynamic field that involves extracting meaningful insights from vast amounts of
data. While powerful algorithms and sophisticated statistical models play a crucial role, data visualization is an equally important aspect of the data science process. Data visualization is the art
of presenting complex data visually, making it easier for analysts and decision-makers to comprehend, interpret, and derive valuable insights. In this blog, we’ll explore the significance of data visualization in data science and its impact on transforming raw data into actionable
knowledge.
1. Simplifying Complexity:
Data science often deals with complex datasets containing multiple variables and relationships. Raw
data in the form of numbers, tables, and figures can be overwhelming and challenging to interpret. Data visualization allows us to represent this data in graphical formats like charts, graphs, and plots,
which are much easier to understand and analyze. Through visual representation, data scientists can identify patterns, trends, and outliers, leading to a better understanding of the underlying data structure.
2. Identifying Patterns and Trends:
Visualizations aid in identifying patterns and trends that might not be apparent in raw data. Scatter
plots, line charts, and heat maps are excellent tools for spotting relationships and correlations
between variables. Data scientists can quickly observe how one variable affects another, leading to
deeper insights and more accurate predictions.
3. Exploring Data Distribution:
Understanding the distribution of data is crucial in data science. Histograms, box plots, and density
plots are valuable tools to visualize data distributions. They help in identifying data skewness, central
tendencies, and variations, which are essential for selecting appropriate statistical methods and
building models that accurately represent the data.
4. Storytelling with Data:
Data visualization enables data scientists to tell compelling stories with data. By presenting
visualizations clearly and engagingly, complex data findings can be effectively communicated to non-
technical stakeholders. Visuals enhance the communication of insights, making it easier for decision-makers to grasp the implications and act upon the findings.
5. Interactive Visualizations:
Interactive data visualizations take the art of data visualization to the next level. Interactive charts
and dashboards allow users to explore data dynamically, drill down into specific details, and adjust
parameters for deeper analysis. These dynamic visuals empower stakeholders to interact with the
data directly, fostering a more participatory and data-driven decision-making process.
6. Detecting Anomalies and Outliers:
Visualizations are excellent tools for detecting anomalies and outliers within datasets. Data points that deviate significantly from the norm can be quickly spotted in scatter plots or box plots.
Identifying these outliers is crucial in data quality assurance and anomaly detection, ensuring data
integrity and accurate analyses.
7. Visualizing Geospatial Data:
For data with geospatial aspects, data visualization becomes even more critical. Maps and geospatial
visualizations allow data scientists to explore data based on geographical location, revealing regional
patterns and spatial relationships. This is particularly valuable in fields like urban planning, logistics,
and environmental studies.
Conclusion:
In the vast landscape of data science, data visualization serves as the bridge between raw data and meaningful insights. By simplifying complexity, identifying patterns and trends, and exploring data distribution, data visualization empowers data scientists to extract valuable knowledge from data. The ability to tell compelling stories with data and present interactive visualizations enhances the communication and understanding of insights across all stakeholders. Moreover, detecting anomalies, and outliers, and visualizing geospatial data add depth and accuracy to the analytical process. As the art of data visualization continues to evolve, it remains a cornerstone of data science, unlocking the full potential of data and driving informed decision-making across industries and domains.
Recent Posts
Recent Comments
- Daniel Ndiema on Data-mining with Python
- Daniel Ndiema on Project 3 Basic Plotting Operations
- Daniel Ndiema on Project 1 Fundamentals of Python
- Daniel Ndiema on Project 2 Data Visualization
- Daniel Ndiema on Introduction to Python Exercises
0 responses on "From Data to Insights: The Art of Data Visualization in Data Science"